Leveraging AI in anti-money laundering initiatives can significantly enhance the detection of suspicious transactions, reduce false positives, and streamline the compliance process for financial institutions. The use of anti-money laundering (AML) software enables compliance specialists to efficiently monitor transactions for suspicious activity that may involve money laundering.
The transaction monitoring systems and tools make it easy for compliance specialists to develop, test and implement the transaction rules that are needed to identify true cases that require investigation and reporting. The transaction monitoring process is enhanced by an artificial intelligence (AI) based transaction monitoring module.
The financial institutions are required to assess their money laundering and terrorist financing risks appropriately, and the applicable regulatory compliance requirements, to develop and implement the right AML solution and systems.

Leveraging AI in Anti-Money Laundering
AI and ML capture transaction data from different linked data sources, such as internal information systems, sanctions data, media, regulatory websites, previously reported suspicious transactions database, etc. The captured data is used to perform meaningful and in-depth analysis for the identification of potential and hidden suspicious patterns or to be red-alerts, considering transaction thresholds, scenarios, and updated risk profiles of relevant customers.
AI and ML assist with profiling clients and customers based on their unique source of funds, demographics, and risks. They also improve the risk assessment process leading to the implementation of more effective risk-based compliance programs, including transaction monitoring and regulatory reporting.
The risk assessment needs to be performed on an ongoing basis, to identify significant risks and accordingly deploy AI based systems that provide real time insights to the big data and multiple data fields, for effective transaction monitoring and investigations.
Data analysis is made easier through the use of AI based AML systems and tools, which further reduce the generation of false positives, and identify suspicious patterns of activity based on defined transaction rules and scenarios.
The advanced rule-builder of AML tools and systems improves risk management practices because transaction monitoring rules may be adjusted to each customer’s risk profile level in accordance with internal compliance and risk management policies.
Use of tools and systems enable performing tests of new transaction rules and scenarios on production data in an integrated sandbox environment, with no impact on day-to-day business activities and operations.
To build, test and deploy matching and screening capabilities it is better for institutions to perform deep testing of possible and defined transaction scenarios and thresholds using the built in AI capabilities of AML transaction monitoring tools and systems.
AML transaction monitoring tools and systems integrate no-code rule-builder and sandbox, and the intuitive and case management. This reduces the high rates of false positive and negative reduction and the use of explainable AI all deliver increased efficiencies across the institution.
An effective AML transaction monitoring tool or system is a crucial part of implementing the risk-based compliance program. AML transaction monitoring tools and software equip compliance specialists with a comprehensive and highly effective view of different interlinked financial transactions, and empower compliance specialists to perform effective reviews based on true match alerts generated for investigation and compliance decisions.
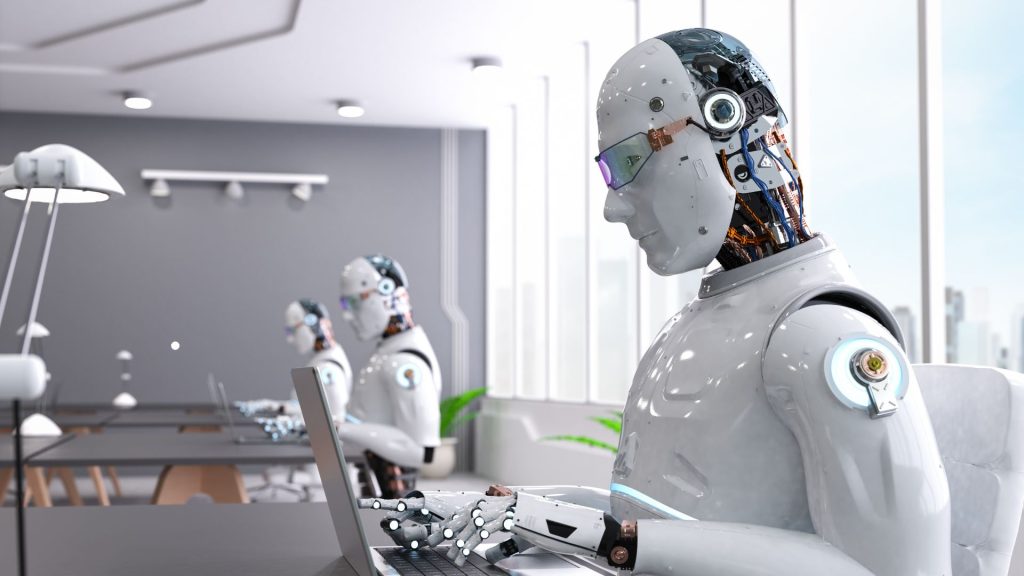
Final Thoughts
The integration of artificial intelligence (AI) and machine learning (ML) in anti-money laundering (AML) systems has revolutionized the field of compliance. These advanced tools enable financial institutions to monitor and analyze vast amounts of transaction data from varied sources with unparalleled depth and precision. With real-time insights into big data, they facilitate the development of risk-based transaction rules tailored to individual customer profiles, reducing false alerts and streamlining investigations.
Furthermore, innovative features like the no-code rule-builder, sandbox environments, and explainable AI foster agility in rule-testing and scenario implementation without disrupting daily operations. In essence, AI-driven AML tools provide compliance specialists with a holistic, efficient view of interlinked financial transactions, ensuring rigorous oversight while enhancing operational efficiency.